Artificial Intelligence in unmanned aircraft systems
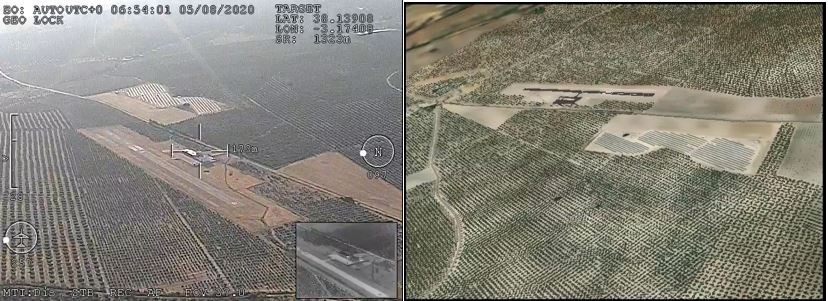
We are currently living in a midst of a technological revolution in which Artificial Intelligence is one of the key pieces of what technology will be like in the future. In this sense, the cinema has already built a mythology around it and created expectations that diverge from the current state of available algorithms. This technology evolution has been ongoing since the Second World War, when the British mathematician Alan Turing laid the foundations for modern computing. In addition, through the article Computing Machinery and Intelligence, he proposed the so-called Turing Test, which suggests a method to discern whether a machine is intelligent on its own. Since then, the advances have followed one after the other until we entered the new millennium, where the combination of cloud computing, big data and the great improvements in machine learning (ML) techniques have allowed an exponential increase in the development and application of the technology of our future.
What is Artificial Intelligence? We know AI as those routines or behaviours of machines or computer operatives that simulate human intelligence. GMV is working to convert this ethereal concept into concrete projects and products, such as the SAFE-Term project, funded by the European Defence Agency (EDA) and developed by GMV in conjunction with AERTEC.
SAFE-Term seeks the safe termination of the flight for an Unmanned Aircraft System (UAS), from the moment the pilot loses control of the aircraft and it suffers an emergency that prevents it from successfully carrying out the contingencies, making it impossible to reach the predefined Flight Termination Areas (FTA). In this case, the UAS must autonomously decide which of the areas that open up in front of it is the most suitable for establishing an Alternative Flight Termination Area (AFTA). This project develops an emergency classification system to deal with this eventuality in MALE (Medium Altitude Long Endurance) UAS, allowing a higher level of safety to be incorporated into their operations.
This project started at the end of 2019 and throughout this time up until now, the team has worked hard to develop a system capable of analysing the environment using computer vision and, through deep neural networks, discerning the safest areas for an emergency landing operation.
The development is divided into three phases, the second of which has already been completed, with remarkable results. The team has succeeded in designing and testing an airborne system, the design of which is marked by the possibility of certification, following guidelines being discussed in various working groups. The aim is for SAFE-Term to be not just another UAS system of the future, but to lay the foundations for the development and incorporation of AI-based systems in the field of aviation.
Among the most noteworthy results of the second phase of the project is the design and implementation of a real avionics system, deployed on hardware that meets all the requirements to be embeddable on an aircraft, which manages to isolate the AI/ML components realistically.
Furthermore, a realistic synthetic simulation environment has been designed and deployed, which allows through the application of Hardware-In-The-Loop (HITL) tests. This allows SAFE-Term to work as it would work if it were on-board an aircraft. The only difference with respect to actual operation is that the data coming in from the Electro-Optical (EO) and Infra-Red (IR) sensors is based on a synthetic database. However, this database has been built on the ATLAS Experimental Flight Centre (Jaén). In this way, maximum realism is sought for the results obtained.
At present, the system resulting from phase two gives an accuracy of 90%, with ratios of less than 5% of false positives, located in non-critical areas, as they are mainly centred on the areas of paths and county roads surrounded by woods. These types of errors do not jeopardise the aircraft's operations in an emergency, as they help to avoid populations. Post-processing means have also been proposed to further reduce the false positive rate by both spatial and temporal consolidation of terrain classification results. These metrics have been obtained with datasets that have been subjected to Gaussian noise and blur treatment, to not only simulate normal operation, but also to understand how they are affected by the most common faults that cameras might suffer. The team can say that the results have been satisfactory and that the system is sufficiently robust within the simulation environment.
For phase 3 of the project, it is planned to optimise the system to improve execution times and results. In the same way, the aim is to train and test SAFE-Term with data obtained from real flight campaigns, in order to complement the process already achieved through training with synthetic data and tests in the simulation environment, with real information from the real world through real sensors, with their deformations and quality deprecation.
At the end of phase 3, the demonstrator can be used as a viable example of a certifiable embeddable system for improving safety in autonomous UAS operations. Much remains to be done, but at GMV we are ready to continue advancing and providing innovative solutions.
Author: Javier Ferrero Micó